RESEARCH ARTICLE
On The Distribution of Partial Sums of Randomly Weighted Powers of Uniform Spacings
Emad-Eldin A. A. Aly1, *
Article Information
Identifiers and Pagination:
Year: 2020Volume: 10
First Page: 1
Last Page: 7
Publisher Id: TOSPJ-10-1
DOI: 10.2174/1876527002010010001
Article History:
Received Date: 02/02/2019Revision Received Date: 06/11/2019
Acceptance Date: 16/11/2019
Electronic publication date: 14/02/2020
Collection year: 2020
open-access license: This is an open access article distributed under the terms of the Creative Commons Attribution 4.0 International Public License (CC-BY 4.0), a copy of which is available at: https://creativecommons.org/licenses/by/4.0/legalcode. This license permits unrestricted use, distribution, and reproduction in any medium, provided the original author and source are credited.
Abstract
Objectives:
To study the asymptotic theory of the randomly wieghted partial sum process of powers of k-spacings from the uniform distribution.
Methods:
Earlier results on the distribution of the uniform incremental randomly weighted sums.
Methods:
Based on theorems on weak and strong approximations of partial sum processes.
Results and conculsions:
Our main contribution is to prove the weak convergence of weighted sum of powers of uniform spacings.
1. INTRODUCTION
Let
be the order statistics of a random sample of size (n-1) from the U(0,1) distribution. Let k=1,2, ... be arbitrary but fixed and assume that n=mk. The U(0,1) k-spacings are defined as

Let X1, X2,... be iidrv with E(Xi)=µ, Var(Xi)=ó2<∞ and common distribution function F(.). Assume that the Xi’s are independent of the Ui's. Define
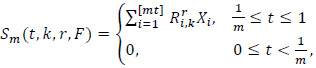
where [s] is the integer part of s and r>0 is fixed.
Looking at Sm (t,k,r,F) of (2) as a weighted partial sum of the X's, Van Assche [1] obtained the exact distribution of S2 (1, 1,1, F). Johnson and Kotz [2] studied some generalizations of Van Assche results. Soltani and Homei [3] considered the finite sample distribution of Sn (1,1,1, F). Soltani and Roozegar [4] considered the finite sample distribution of a case similar to Sm (1,k,1, F) in which the spacings (1) are not equally spaced. It is interesting to note that Sm (t,k,r, F) of (2) is also a randomly weighted partial sum of powers of k-spacings from the U(0,1) distribution.
Here, we will obtain the asymptotic distribution of the stochastic process
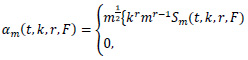
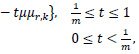
where

and Γ(.) is the gamma function.
The motivations and justifications of this work are given next. First, as noted by Johnson and Kotz [2], S2 (1,1,1, F) is a random mixture of distributions and as such it has numerous applications in Sociology and in Biology. Second, the asymptotic theory of Sm (t,k,r, F) is a generalization of important results of Kimball [5], Darling [6], LeCam [7], Sethuraman and Rao [8], Koziol [9], Aly [10] and Aly [11] for sums of powers of spacings from the U(0,1) distribution. Finally, we solve the open problem of proving the asymptotic normality of Sm (1,k,1, F) proposed by Soltani and Roozegar [4].
2. METHODS
2.1. The asymptotic distribution of αm (., k,r, F)
Let Y1,Y2,... be iidrv with the exponential distribution with mean 1 which are independent of the Xi's. By Proposition 13.15 of Breiman [12] we have for each n,


Hence, for each m,

where for 1≤i≤m,

are iid Gamma (k,1) random variables. Hence, for each m

and

where
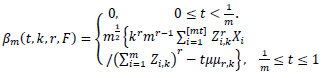
Let µl,k be as in (4). Note that
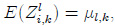
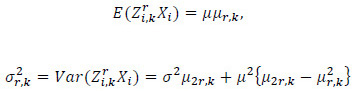
and

The following Theorem will be needed in the sequel.
Theorem A. There exists a probability space on which a two-dimensional Wiener process
is defined such that


where E W (s)=0, and

Theorem A follows from the results of Einmahl [13], Zaitsev [14] and Götze and Zaitsev [15].
The main result of this paper is the following Theorem.
Theorem 1. On some probability space, there exists a sequence of mean zero Gaussian processes Γm(t, k, r, F), 0≤t≤1 such that

where
for each m, and

Theorem 1 follows from (6) and the following Theorem.
Theorem 2. On the probability space of Theorem A,


where W (.) is as in (9).
Proof of Theorem 2: We will only prove here the case when E(X)=µ≠0. The case when µ=0 is straightforward and can be looked at as a special case of the case µ≠0. Note that
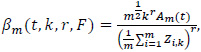
where
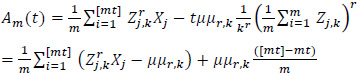

It is clear that, uniformly in t, 0≤t≤1,
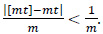
By (9), (15) and (16) we have, uniformly in t,0≤t≤1,

By Lemma 1.1.1 of Csörgö and Révész [17] we have, uniformly in t,0≤t≤1,

By (17) and (18) we have, uniformly in t,0≤t≤1,



By the LIL


By (14), (19) and (20) we have, uniformly in t,0≤t≤1,



This proves (13).
Corollary 1. By (4), (8) and (12),

where

W(.) is a Wiener process, B(.) is a Brownian bridge and W(.) and B(.) are independent.
Corollary 2. By (11) and (21) we have, as m→∞,

and, in particular,

Some special cases of (22) and (23) are given . For r=1 and k≥1,

and


and

where

3. RESULTS
In this section, we will use the same notation of Section 1
3.1. The scaled sum case
Define

and

We can prove that

where

(W1(.),W2(.),W3(.))t is a mean zero Gaussian vector with covariance (t Λ s) ∑ 1 and
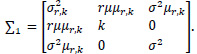
Let

We can show that

where W(.) is a Brownian Motion and B(.) is a Brownian bridge and W(.) and B(.) are independent. Consequently,

When r=1,k≥1

When r>0,k=1

3.2. The Centered Sum Process
Let
and define
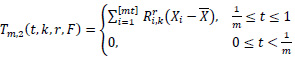
and

We can prove that

where

(W1(.), W2(.), W3(.))t is a mean zero Gaussian vector with covariance (t Λ s) ∑2 and
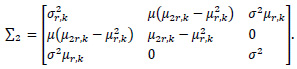
We can show that

where W(.) is a Brownian Motion and B (.) is a Brownian bridge and W(.) and B(.) are independent. Consequently,

When r=1,k≥1

When r> 0,k=1

3.3. The Renewal Process
For simplicity, we will consider the case of r=1. Define
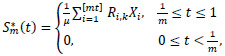

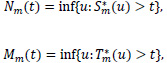
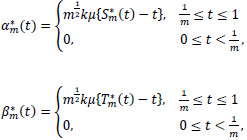

and

By (5), for each m

Note that (see (3))

and hence, by Theorem 1

where Γm (., k, 1, F) is as in (11).
Theorem 3. On the probability space of Theorem A,

where

and W(.) is as in (9).
Theorem 3 follows directly from (24) and the following Theorem.
Theorem 4. On the probability space of Theorem A,

where Γm(t) is as in (25).
Proof: By (7),

Note that

Hence

where


and

By Theorem 2 and the LIL for Wiener processes,
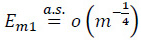
and

By a Lemma of Horváth [18]

and hence

By the proof of Step 5 of Horváth [18] and Theorem 2 we can show that
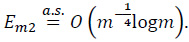
As to Em3,

where

and

By (28) and Lemma 1.1.1 of Csörgö and Révész [17] we have, uniformly in t,0≤t≤1,



By (28) and the LIL for Wiener processes,

By (30)-(32),

By (26)-(33) we obtain Theorem 4.
4. THE RANDOM VECTOR CASE
Let X1, X2,... be iid random vectors with
and
Assume that the Ui's and the Ri,ks are same as in Section 1 and are independent of X1, X2,... Define
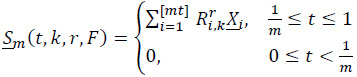
and
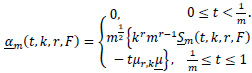
Theorem 5 is a generalization of Theorem 1.
Theorem 5. On some probability space, there exists a mean zero sequence of Gaussian processes
such that

where, for each m,



and

Corollary 1 *. By (11) and (21) we have, as m→∞,

and, in particular,

where

Particular cases of Corollary 1* are given next.
For r = 1 and k ≥ 1,



and

For r > 0 and k = 1,




and

where


CONCLUSION
We proved the weak convergence of a stochastic process defined in terms of partial sums of randomly weighted powers of uniform spacings. The asymptotic results of several important generalizations and special cases are given.
CONSENT FOR PUBLICATION
Not applicable.
AVAILABILITY OF DATA AND MATERIALS
Not applicable.
FUNDING
None.
CONFLICT OF INTEREST
The author declare no conflict of interest, financial or otherwise.
ACKNOWLEDGEMENTS
Declared none.